"A spiking neural network model for proprioception of limb kinematics in insect locomotion", by van der Veen et al. 2025.
https://www.biorxiv.org/content/10.1101/2024.09.27.615365v1.full
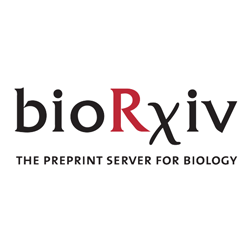
"A spiking neural network model for proprioception of limb kinematics in insect locomotion", by van der Veen et al. 2025.
https://www.biorxiv.org/content/10.1101/2024.09.27.615365v1.full
Wtf how did I roll so ridiculously bad in proprioception? Constantly walking into things, smashing extremities on everything, knocking things over more than a cat with zoomies...
#Proprioception et équilibre : notre « sixième sens » varie d’un individu à l’autre
I absolutely struggle with touch screen keyboards. Whether a 6" screen or a 12" screen, doesn't matter. It's probably related to my top-tier klutziness since childhood. I can't ride a bike, can't put my pants on without leaning against a wall, etc. It's bad. I'd never pass a field sobriety test.
This is why one may see me bust out a Bluetooth mechanical keyboard from time to time for my tablet.
It's also why my posts and comments on here are often lacking punctuation or capitalization
What's on my mind?
Disappointment and Disillusionment!
- Invested 7 yrs in #GoPiGo3 #robot
- Created #ROS2 nodes for #proprioception, #odometry, #LIDAR, #docking, #tts #mapping, #navigation, #simulation, #ObjectRecognition, and #LifeLogging
- My robot cannot safely and reliably navigate in my complex home environment.
- No slower turns, no inflation value can fix
I'm done, destroyed, no strength left for "one more try". GoPi5Go-Dave is on the shelf with other "reached its limit" robots.
Passer une partie de son temps à se tromper de bouton sur la manette en jouant à Zelda tears of Kingdom, c'est appuyer sur Y et exploser un truc que t'as mis 3 plombes à fabriquer, alors que tu voulais cliquer sur X pour sauter dessus, mais que dans le stress parce qu'il bouge, tu t'emmêles les doigts et le cerveau
I don't understand what I'm feeling. Depression? Exhaustion? Heartbreak? Maybe even fear? Dissatisfaction? Something just isn't quite right. I'm not sure what to do about it because I don't know what it is. It's so subtle I can hardly hear it but it's constantly there. Been going on for days, I think even weeks.
#mentalhealth #depression #exhaustion #love #proprioception #alexithymia #neurodivergent #actuallyautistic #scared
Buttons, knobs, and sliders, oh my! I'm so glad that the #design pendulum is moving away from flat screens for everything. We shouldn't have to look at a screen to turn up the AC in the car. And washing machines? #cmon #HumanCenteredDesign #proprioception https://www.wsj.com/tech/personal-tech/touch-screens-are-over-even-apple-is-bringing-back-buttons-86fb9ea8?st=oiJUVd&reflink=desktopwebshare_permalink
My #proprioception is shot to shit because I'm tired after preparing all day for a job interview that wasn't of any actual fucking use so of course I tripped on a step & took a stripe off my big toe while I was on the way to get a beer to cheer myself up. #grumpy
The power of #proprioception: how to improve your ‘sixth sense’ – and become healthier and happier
It is our physical sense of where we are in space and is essential to our quality of life as we age. Here are simple, everyday ways to test and train it
Dites, sur Insta j'ai plein de pubs pour quelque chose qui s'appelle du #yoga somatique.
J'ai pas de problème de poids mais je me demande en tant qu'#autiste avec des problèmes de #proprioception et d'#interoception si c'est différent du yoga "classique" et si ça peut m'aider davantage.
Des retours d'expérience sur le #YogaSomatique, que vous soyez #actuallyAutisticFR ou pas ?
I'm thinking about #proprioception today & how it might relate to my intense dislike of dancing.
I understand that others like dancing & think I can understand why (although it's a very abstract understanding). I even have a version of it in my head where I enjoy the rhythm of it but the moment there's an attempt to translate that from thought to physical act my brain goes from "this is fun" to "I absolutely hate this, make it stop".
Does anyone else have this?
#ActuallyAutistic
Honestly, I'd love to solely work out at home, but between parenting and #ADHD, it's hard to organise my personal time and space.
I also do better with body doubling, and my poor #proprioception means I often need someone there in person to check and correct my form. It's the only way I managed to make progress back when I started doing yoga.
I'll be giving a talk at #SfN23 about my past, present and future research into how we use #proprioception to produce robust #locomotion. If this is your jam, join me this Sunday at 11 am!
A brief perspective by myself and Niccolò Zampieri on #proprioception: what it is, what we know about it and what tools we can use to explore what is not yet known.
authors.elsevier.com/a/1i1RpcQbJ7X7c
@DrTCombs the #SpatialEducation of #children requires activity and interaction with the natural and #BuiltEnvironment.
#Proprioception develops through the use of the body as vessel, tool and means to inhabit space via sensory input and increasingly appropriate response to stimuli.
So, that is a fairly concerning institutional approach.
Are you in Fukuoka for #ISB2023? Come and find me to talk #locomotion, #proprioception and #musclesyneRgies! Thursday 3rd at 11:00, Oral session 69, Motor Control 2, room 3.
Does visual experience influence arm #proprioception and its lateralization? Evidence from passive matching performance in #congenitally-#blind and #sighted adults https://www.sciencedirect.com/science/article/abs/pii/S030439402300294X