#statstab #334 Workflow Techniques for the Robust Use of Bayes Factors
Thoughts: "We outline a Bayes factor workflow that researchers can use to study whether Bayes factors are robust for their individual analysis"
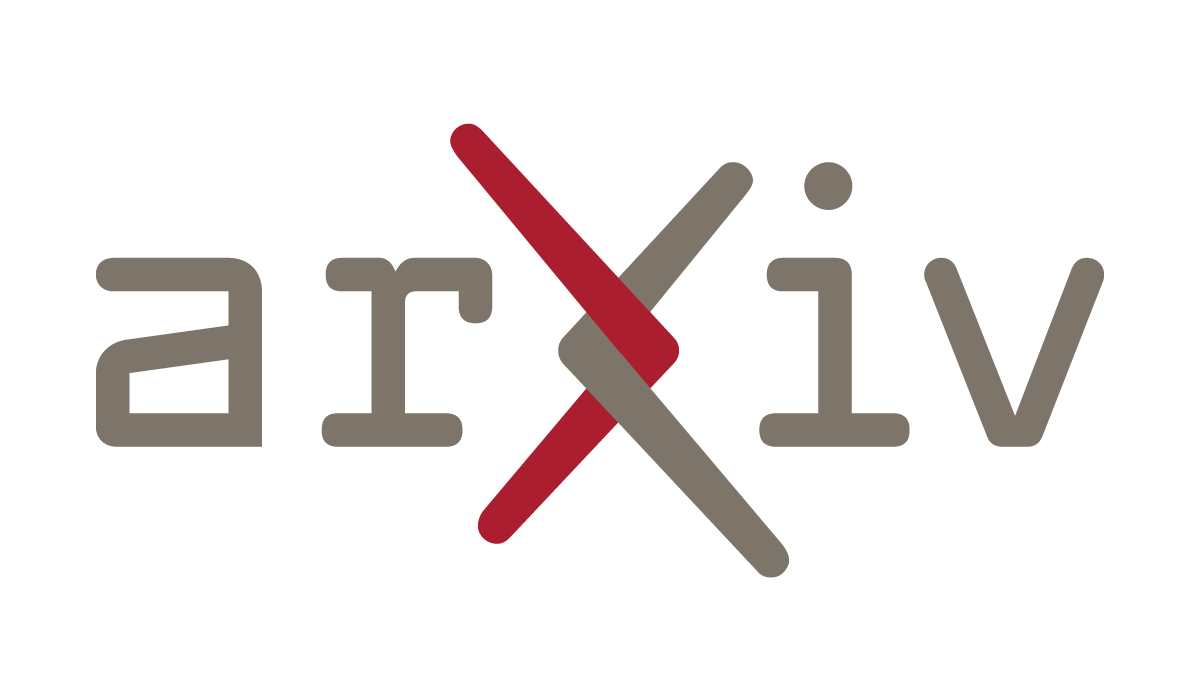
#statstab #334 Workflow Techniques for the Robust Use of Bayes Factors
Thoughts: "We outline a Bayes factor workflow that researchers can use to study whether Bayes factors are robust for their individual analysis"
I am trying to get a better sense of the literature on frequentist properties of Bayesian posterior distributions - both empirical and theoretical. Do you have any recommendations on stuff I should not miss? #stats #bayesian #LitReviews
'Determine the Number of States in Hidden Markov Models via Marginal Likelihood', by Yang Chen, Cheng-Der Fuh, Chu-Lan Michael Kao.
http://jmlr.org/papers/v26/23-0343.html
#markov #bayesian #likelihood
Die schwierige Bergung der Luxusjacht "Bayesian"
Eine riesige Luxusjacht sinkt im Mittelmeer: Das Unglück der "Bayesian", bei dem sieben Menschen starben, sorgte 2024 für Schlagzeilen und Spekulationen. Nun soll das Wrack geborgen werden, doch die Aktion verzögert sich.
I'd like to move the Git repo for my R protopackage ("Inferno") from GitHub to Codeberg. I was wondering if Codeberg offers (even paying of course) something similar to GitHub pages, by which I mean something like this: https://pglpm.github.io/inferno
From what I understand, the Codeberg pages <https://docs.codeberg.org/codeberg-pages> should be something equivalent – but I'm not fully sure. Is that correct? And does anyone have examples of Codeberg pages of R packages, just to see their functionality? I didn't manage to find any examples.
Cheers!
Environmental & Anthropogenic Influences On Fire Patterns In Tropical Dry Deciduous Forests
--
https://doi.org/10.1038/s41598-025-98051-7 <-- shared paper
--
#GIS #spatial #mapping #wildfire #fire #busgfire #nvironmental #anthropogenic #influences #humanimpacts #deciduous #forests #firepatterns #spatialanalysis #tropical #ecosystems #management #tree #vegetation #spatiotemporal #hotspots #india #Satpura #tiger #reserve #ecogeography #geography #factors #parameters #drivers #temperature #precipitation #rainfall #model #modeling #bayesian #framework #slope #water #hydrology #infrastructure #mitigation #monitoring #preparedness #forestfires
#statstab #328 How to Assess Task Reliability using Bayesian Mixed Models
by @Dom_Makowski
Thoughts: Nice walkthrough using {brms}, with code, data gen, and plots.
#r #bayesian #mixedeffects #reliability #brms
https://realitybending.github.io/post/2024-03-18-signaltonoisemixed/
Observations Reveal Changing Coastal Storm Extremes Around The United States
--
https://doi.org/10.1038/s41558-025-02315-z <-- shared paper
--
#GIS #spatial #mapping #extremeweather #coast #coastal #spatialanalysis #spatiotemporal #model #modeling #communities #publicsafety #climatechange #stormsurge #USA #flood #flooding #risk #hazard #damage #infrastructure #cost #economics #mitigation #insurance #sealevel #SLR #sealevelrise #Bayesian #hierarchical #framework #tideguage #tide #tidal #water #hydrology #hydrography #extremes #intensity #monitoring
'DAGs as Minimal I-maps for the Induced Models of Causal Bayesian Networks under Conditioning', by Xiangdong Xie, Jiahua Guo, Yi Sun.
http://jmlr.org/papers/v26/23-0002.html
#inference #causal #bayesian
#statstab #319 Small Sample Size Solutions [book]
Thoughts: This should just be the default text for psychologists, as most research fits the "small sample" label.
#smallsample #book #guide #bayesian #permutation #sem #metaanalysis #nof1 #missingdata
PyMC is in Google Summer of Code 2025!
We're excited to be part of #GSoC2025 under @NumFOCUS If you're passionate about #Bayesian stats & #OpenSource, this is your chance to contribute to #PyMC!
Deadline: April 8, 18:00 UTC
Apply now: https://www.pymc.io/blog/blog_gsoc_2025_announcement.html
New paper! We develop a #Bayesian statistical model to better predict future publishing counts by an institution w/ a particular publisher and the associated #APCs. This is important because any #OpenAccess negotiation depends heavily on the expected publication output.
It's important to emphasize that "realistic-looking" data does *not* mean "realistic" data – especially high-dimensional data (unfortunately that post doesn't warn against this).
If one had an algorithm that generated realistic data for a given inference problem, it would mean that that inference problem had been solved. So: for educational purposes, why not. But for validation-like purposes, use with uttermost caution and at your own peril.
The bayesEO package provides a Bayesian approach to post-processing ML-generated images. It refines class probabilities, removes outliers, and improves labeling for more accurate classification.
Happy Birthday, Laplace!
One of the first to use Bayesian probability theory in the modern way!
"One sees in this essay that the theory of probabilities is basically only common sense reduced to a calculus. It makes one estimate accurately what right-minded people feel by a sort of instinct, often without being able to give a reason for it. It leaves nothing arbitrary in the choice of opinions and of making up one's mind, every time one is able, by this means, to determine the most advantageous choice. Thereby, it becomes the most happy supplement to ignorance and to the weakness of the human mind. If one considers the analytical methods to which this theory has given rise, the truth of the principles that serve as the groundwork, the subtle and delicate logic needed to use them in the solution of the problems, the public-benefit businesses that depend on it, and the extension that it has received and may still receive from its application to the most important questions of natural philosophy and the moral sciences; if one observes also that even in matters which cannot be handled by the calculus, it gives the best rough estimates to guide us in our judgements, and that it teaches us to guard ourselves from the illusions which often mislead us, one will see that there is no science at all more worthy of our consideration, and that it would be a most useful part of the system of public education."
*Philosophical Essay on Probabilities*, 1814 <https://doi.org/10.1007/978-1-4612-4184-3>
#statstab #299 The role of "max_treedepth" in No-U-Turn?
Thoughts: Once you start using more complex models you will run into issues at some point; this is one; good solution guide.
#brms #bayesian #modeling #stats #issues #solutions #stan #forum
https://discourse.mc-stan.org/t/the-role-of-max-treedepth-in-no-u-turn/24155
After a long collaboration with @martinbiehl, @mc and @Nathaniel I’m excited to share the first of (hopefully) many outputs:
“A Bayesian Interpretation of the Internal Model Principle”
https://arxiv.org/abs/2503.00511.
This work combines ideas from control theory, applied #categorytheory and #Bayesian reasoning, with ramifications for #cognitive science, #AI/#ML, #ALife and biology to be further explored in the future.
In these fields, we come across ideas of “models”, “internal models”, “world models”, etc. but it is hard to find formal definitions, and when one does, they usually aren’t general enough to cover all the aspects these different fields consider important.
In this work, we focus on two specific definitions of models, and show their connections. One is inspired by work in control theory, and one comes from Bayesian inference/filtering for cognitive science, AI and ALife, and is formalised with Markov categories.
In the first part, we review and reformulate the “internal model principle” from control theory (at least, one of its versions) in a more modern language heavily inspired by categorical systems theory (https://www.davidjaz.com/Papers/DynamicalBook.pdf, https://github.com/mattecapu/categorical-systems-theory/blob/master/main.pdf).
6/ Thus we built upon this #Bayesian framework (https://doi.org/10.1038/s41467-021-24149-x) to infer each pairwise interaction in individual-based #networks accounting for sampling effort and the inherent stochasticity of field data
#statstab #293 The Bayesian Bootstrap
Thoughts: I need to think more on where bootstrapping makes sense in a bayesian setting. But here's a tutorial.
#stats #bayesian #bayes #bootstrap #resampling
https://towardsdatascience.com/the-bayesian-bootstrap-6ca4a1d45148/