Using Integer Addition to Approximate Float Multiplication - Once the domain of esoteric scientific and business computing, floating point calc... - https://hackaday.com/2025/04/10/using-integer-addition-to-approximate-float-multiplication/ #softwaredevelopment #softwarehacks #floatingpoint #fpu #gpu
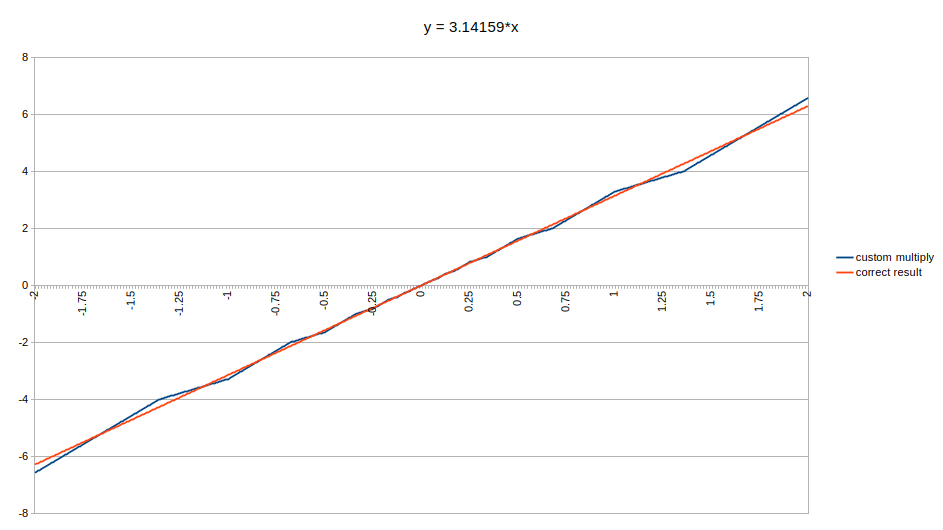